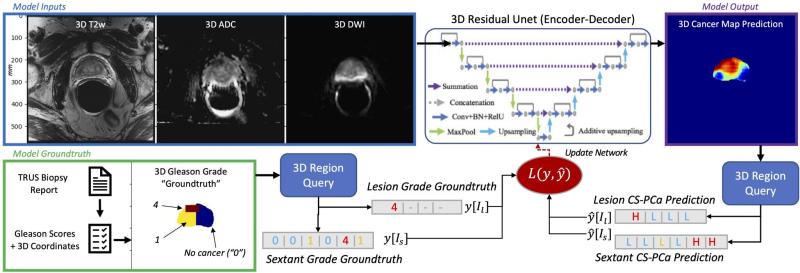
Pictured above: Deep Learning model architecture utilizing 3D T2WI, ADC, and DWI MRI inputs to produce a 3D cancer risk map. The training uses a loss function for mixed strong (targeted biopsy) and weak (systematic biopsy) results.
The UCSF Center for Intelligent Imaging (ci2) and the Duke Center for Artificial Intelligence in Radiology (DAIR) are collaborating to improve the detection of clinically significant prostate cancer from prostate MRI.
“Prostate cancer presents a conundrum,” says Kirti Magudia, MD, Ph.D., currently an assistant professor in the Abdominal Imaging Division at Duke Radiology and formerly a clinical and research fellow at UCSF Radiology. “Although 11% of men will develop prostate cancer in their lifetime, the large majority of these will have localized disease with 100% survival and will not die from their prostate cancer. The key is identifying those men with aggressive prostate cancer.”
Given so many men are identified at risk for prostate cancer by PSA screening, numerous men undergo a prostate biopsy to determine if they have prostate cancer and if so, whether it is clinically significant (i.e., aggressive). Prostate MRI has the potential to risk-stratify patients with the promise of decreasing unnecessary biopsies.
Analyzing prostate MRI images with artificial intelligence (AI) has great potential to improve the diagnosis of clinically significant prostate cancer. In particular, deep learning methods based on convolutional neural networks have the power to extract complex correlations from medical images to improve the prediction of aggressive disease as well as improve reliability and speed of interpretation.
Large, curated image datasets that capture the range of prostate cancer are key for high-performance deep learning methods. To this end, UCSF ci2 and Duke DAIR plan to join forces, combining their data, clinical experience, and deep learning expertise. “Working across institutions has the potential to provide large improvements in the algorithms, and also means they will generalize better to prostate MRI acquired at different institutions and on different scanners,” says Peder Larson, Ph.D., UCSF ci2 member and associate professor at UCSF Radiology. “Multi-site collaborations are the next frontier for improving medical imaging AI methods.”
During her fellowship at UCSF, Dr. Magudia assembled a dataset of almost 1000 patients who underwent prostate MRI followed by both targeted MRI/ultrasound fusion and nontargeted systematic prostate biopsies. Abhejit Rajagopal, Ph.D., a postdoctoral fellow in Dr. Larson’s group, developed a deep learning model trained on this unique data that creates a prostate cancer risk map. These maps clearly show promise in identifying areas of concern in the prostate gland and could form the basis for improved assessment of aggressive cancers.
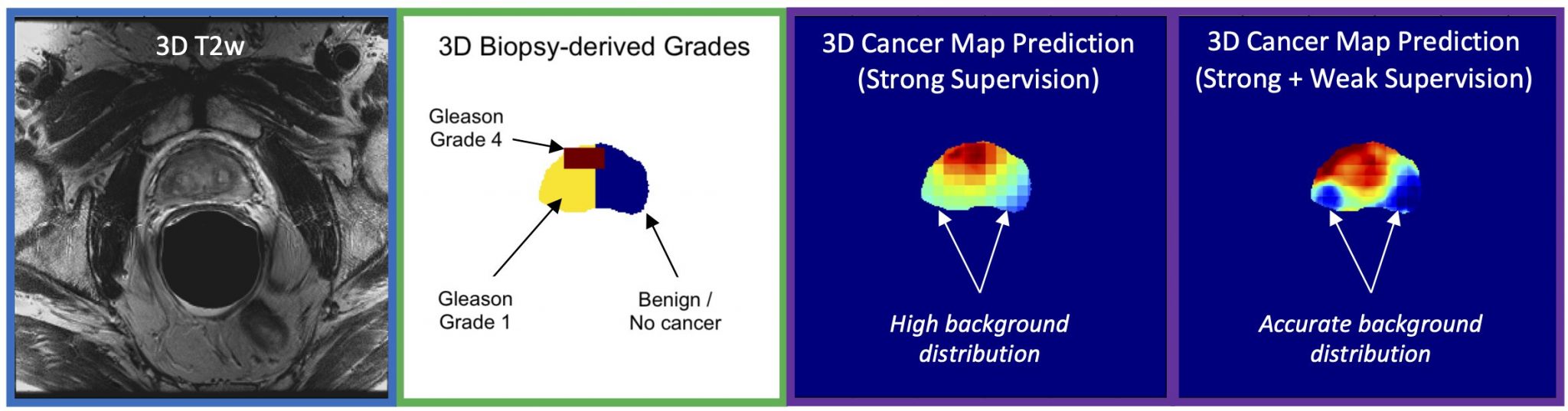